Navigating the Data Analytics Landscape: What Every Product Owner Needs to Know
The next five years will see significant advancements in data analytics, particularly in the areas of AI-driven insights and real-time data processing. Tools that can seamlessly integrate with AI and machine learning algorithms will lead the market. Moreover, the rise of edge computing will require data analytics tools that can operate efficiently in decentralized environments.
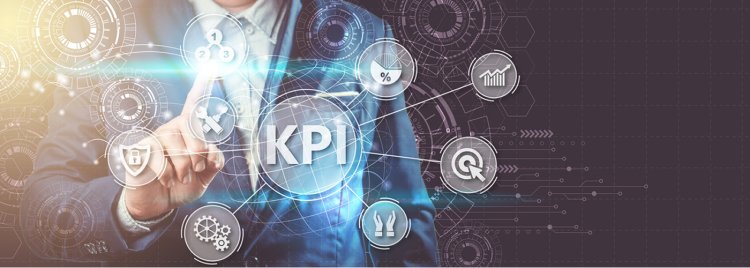
In today's data-driven world, the role of a Product Owner has evolved to become increasingly intertwined with data analytics. Understanding the landscape of tools and technologies available is crucial for those who wish to stay ahead in their respective industries. With the experience of having been working with an intelligence and operational data analytics platform capturing data from different devices such as industrial printers, mobile computers and scanners, I’ve learned why the rapid shift towards data-centric decision-making.
I’ve always been driven by a passion for innovation. This same passion fuels my interest in exploring the best tools for data analytics—a subject that is not just relevant but essential for Product Owners today.
In this blog, I’ll be diving into the world of data analytics tools, offering a detailed comparative analysis of the best options available for Product Owners. We’ll explore the fundamental terminology you need to know, the key knowledge areas to focus on, and how these tools integrate with cloud-based microservices, yes microservices again, I learned that this is a key element for us to monitor. Whether you’re in manufacturing, healthcare, or banking, this guide will provide insights into the major market players, common use cases, challenges, and the future trajectory of data analytics. Let’s embark on this journey to empower Product Owners to make data-driven decisions that drive success.
Data analytics involves the systematic computational analysis of data or statistics. It’s used across industries to make informed decisions, predict trends, and optimize operations. As a Product Owner, knowing the basics—descriptive, diagnostic, predictive, and prescriptive analytics—is essential. A Product Owner should have a grasp of basic statistical concepts, data processing, and the importance of clean, structured data. Familiarity with data governance and data ethics is also important in today’s data-driven world.
Each type of data analytics serves a unique purpose:
- Descriptive Analytics: Focuses on what has happened in the past using data aggregation and mining techniques.
- Diagnostic Analytics: Explains why something happened through drill-down, data discovery, and correlations.
- Predictive Analytics: Uses statistical models and machine learning techniques to predict future outcomes.
- Prescriptive Analytics: Suggests actions to take for optimal outcomes based on predictive analytics.
Product Owners need to be familiar with the tools that cater to each type of analytics. Here’s a comparative analysis:
Tool | Type of Analytics | Key Features | Market Competitors |
---|---|---|---|
Sisense | All Types | Full-stack data platform, AI integration | Tableau, Power BI |
Tableau | Descriptive, Diagnostic | User-friendly dashboards, strong visualizations | Qlik Sense |
Power BI | All Types | Seamless Microsoft integration, extensive capabilities | Looker, Domo |
Qlik Sense | Descriptive, Diagnostic | Associative engine, self-service analytics | Tableau, Sisense |
Looker | Predictive, Prescriptive | Embedded analytics, modern data architecture | Power BI, Sisense |
In the fast-paced world of data analytics, Product Owners must familiarize themselves with a variety of tools tailored to different industry needs. Whether you're working in healthcare, transportation, or agriculture, selecting the right tool is crucial for leveraging data effectively. Tools like Sisense, Tableau, and Power BI have become staples in the industry, each offering unique strengths in terms of visualization, scalability, and integration capabilities.
To make informed decisions, Product Owners need a clear understanding of how these tools stack up against each other. Below is a comparative chart that breaks down key features across several leading platforms:
Tool | Strengths | Weaknesses | Best Suited For |
---|---|---|---|
Sisense | Robust integration, scalability | Steep learning curve | Manufacturing, Healthcare |
Tableau | Advanced visualization, user-friendly | Limited customization | Retail, Banking |
Power BI | Seamless Microsoft ecosystem integration | Performance issues with large data | Transportation, Logistics |
Looker | Strong in data modeling | Expensive | Technology, Startups |
Qlik Sense | Real-time analytics | Complex setup | Financial Services, Agriculture |
This chart highlights how different tools cater to specific industry needs and challenges. For instance, Sisense’s robustness in handling large datasets makes it a go-to for manufacturing and healthcare, whereas Tableau’s user-friendly interface is ideal for retail and banking sectors.
To effectively manage data analytics projects, Product Owners need to be well-versed in key terminology:
- Data Warehousing: The process of collecting and managing data from varied sources to provide meaningful business insights.
- ETL (Extract, Transform, Load): A data processing system used to consolidate data from multiple sources.
- Data Lake: A storage repository that holds a vast amount of raw data in its native format until it is needed.
- Predictive Analytics: The use of statistical algorithms and machine learning techniques to identify the likelihood of future outcomes.
- Operational Intelligence (OI): Real-time dynamic business analytics that delivers visibility and insight into data, streaming events, and business operations.
One of the critical challenges for Product Owners is aligning data analytics with the development of cloud-based microservices. Microservices architecture requires tools that can seamlessly integrate with cloud environments, ensuring that data flows efficiently between services. Platforms like Sisense and Looker offer strong API support, enabling integration with microservices in real-time, which is crucial for operational intelligence.
Cloud-based microservices architecture allows for scalable and efficient data processing. Understanding how data analytics integrates with microservices can help in designing products that are both robust and flexible.
The data analytics market is fiercely competitive, with several key players dominating different segments. Sisense leads in manufacturing and healthcare, thanks to its ability to handle complex data integrations and provide real-time insights. Tableau and Power BI are strong contenders in retail and financial services due to their superior data visualization capabilities. Meanwhile, Looker and Qlik Sense have made significant inroads in the technology and startup sectors, offering robust data modeling and real-time analytics, respectively.
Data analytics tools are being deployed across a wide range of industries, each with unique use cases:
- Manufacturing: Predictive maintenance and quality control through real-time analytics.
- Healthcare: Patient data management and predictive analytics for disease outbreaks.
- Transportation & Logistics: Route optimization and real-time fleet management.
- Agriculture: Precision farming and yield prediction using big data.
- Banking: Fraud detection and personalized financial services.
- Retail: Customer behavior analysis and inventory management.
Challenges in data analytics often revolve around data integration, scalability, and ensuring real-time insights. For instance, manufacturing companies might struggle with integrating data from legacy systems with modern analytics tools. Similarly, healthcare organizations face the challenge of maintaining data privacy while leveraging analytics for patient care. Tools like Sisense and Looker have developed solutions to these challenges, offering robust data integration capabilities and compliance with privacy regulations.
The next five years will see significant advancements in data analytics, particularly in the areas of AI-driven insights and real-time data processing. Tools that can seamlessly integrate with AI and machine learning algorithms will lead the market. Moreover, the rise of edge computing will require data analytics tools that can operate efficiently in decentralized environments.
When selecting a platform to support data analytics initiatives, Product Owners should consider factors such as scalability, integration capabilities, and cost. Platforms like Microsoft Azure and AWS provide comprehensive support for data analytics, offering everything from data storage to machine learning tools. Google Cloud Platform is another strong contender, particularly for startups and technology companies.
Product Owners must work closely with data scientists, development teams, and architects to ensure that data analytics initiatives align with overall business goals. This collaboration is particularly important when dealing with SaaS solutions focused on operational intelligence. Onboarding teams effectively is crucial for optimizing the use of these tools and ensuring that all stakeholders are aligned.
As data continues to drive decision-making across industries, Product Owners must stay informed about the latest tools and trends in data analytics. The ability to understand and leverage these tools can significantly impact a product's success in the market. With the right knowledge and resources, Product Owners can navigate the complexities of data analytics, ensuring that their teams are equipped to deliver real-time insights and drive business growth.
Thank you for taking the time to read this in-depth analysis of the best tools for Product Owners to use in Data Analytics. I hope you found the information valuable and insightful for your journey as a Product Owner. I would love to hear your thoughts, experiences, and any additional insights you might have. Please feel free to share this article on your social networks, leave a comment with your suggestions or questions, and click 'like' if you enjoyed the read. If you’d like to discuss these ideas further or explore how these tools can be tailored to your specific needs, don't hesitate to reach out via email. Let's keep the conversation going and continue learning together!
Gartner. (2024). Cloud-Based Data Analytics Platforms: Market Forecast and Trends. Retrieved from Gartner. IDC. (2023). The Growth of Data Analytics Tools in Manufacturing. International Data Corporation. Forrester. (2023). Challenges in Implementing Data Analytics Tools. Forrester Research. McKinsey. (2023). Data Analytics Tools: Industry-Wise Usage and Preferences. McKinsey & Company. AWS. (2024). Integrating Data Analytics into Cloud-Based Microservices. Amazon Web Services. Harvard Business Review. (2024). Data-Driven Decision Making for Product Owners. Harvard Business Publishing. Deloitte. (2023). The Impact of Data Analytics on Business Growth in Retail. Deloitte Insights. PwC. (2024). Complexity vs. Adoption: The Balance in Data Analytics Tools. PricewaterhouseCoopers. Google Cloud. (2024). BigQuery for Data Analytics: Best Practices and Use Cases. Google Cloud. Microsoft Azure. (2024). Synapse Analytics: Powering Data-Driven Decision Making. Microsoft Corporation.
What's Your Reaction?
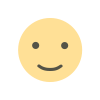
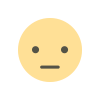

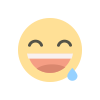
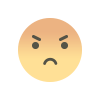
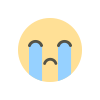
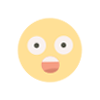